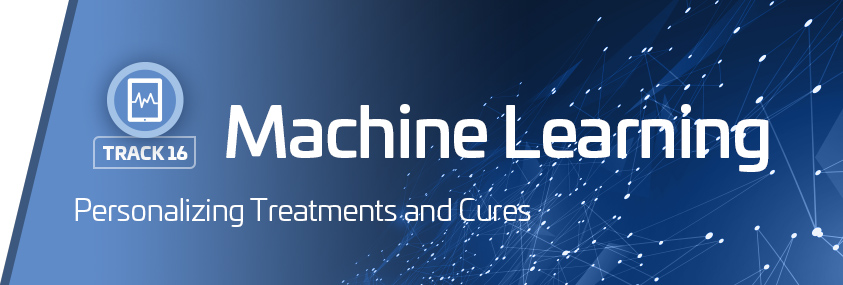
The role of computer science in modeling cells, analyzing and mapping data networks, and incorporating clinical and pathological data to determine how diseases arise from mutations is becoming more important in genomic medicine. We need to understand
where the disease starts and how artificial intelligence delivers genes and pathways for drug targets and diagnostics. Track 16 explores case studies that apply deep learning, machine learning, and artificial intelligence to medicine. We will discuss
data curation techniques, text mining approaches, and statistical analytics that utilize deep machine learning to support AI efforts. This will help to integrate omics approaches to discover disease or drug response pathways and identify personalized
and focused treatments and cures.
Tuesday, May 15
7:00 am Workshop Registration Open (Commonwealth Hall) and Morning Coffee (Foyer)
8:00 – 11:30 Recommended Morning Pre-Conference Workshops*
W3. D2K, Transforming Data to Knowledge with Cloud, IoT & Machine learning (AI) – Part I
12:30 – 4:00 pm Recommended Afternoon Pre-Conference Workshops*
W9. D2K, Transforming Data to Knowledge with Cloud, IoT & Machine learning (AI) – Part II
* Separate registration required.
2:00 – 6:30 Main Conference Registration Open (Commonwealth Hall)
4:00 PLENARY KEYNOTE SESSION (Amphitheater & Harborview 2)
5:00 – 7:00 Welcome Reception in the Exhibit Hall with Poster Viewing (Commonwealth Hall)
Wednesday, May 16
7:00 am Registration Open (Commonwealth Hall) and Morning Coffee (Foyer)
8:00 PLENARY KEYNOTE SESSION (Amphitheater & Harborview 2)
9:45 Coffee Break in the Exhibit Hall with Poster Viewing (Commonwealth Hall)
10:50 Chairperson’s Remarks
Ted Slater, Global Head, Scientific AI & Analytics, Cray Inc.
11:00 KEYNOTE PRESENTATION: Can AI Beat Cancer?
Jay (Marty) Tenenbaum, PhD, Founder and Chairman, Cancer Commons
AI can beat go and drive cars, but can it beat cancer? Every year, many thousands of cancer patients die unnecessarily because their doctors do not know the optimal way to treat them with currently available therapies. Physicians and patients alike
struggle with information overload and conflicting expert opinions in making treatment decisions. Moreover, effective treatments increasingly involve intelligently designed, individually tailored, sequences and combinations, and there are far
more plausible multi-drug regimens than can be efficiently tested in clinical trials. AI can help by connecting physicians and patients to the right information at the right time, and by planning and coordinating the thousands of formal and informal
treatment experiments that take place daily in oncology, to optimize individual outcomes and maximize collective knowledge. We will describe a developing global collaboration to realize this bold vision, involving leading oncologists, cancer and
data scientists, and AI experts from both academia and industry, and discuss opportunities for all to participate.
12:00 pm Empowering Medical Practitioners to Train Models for AI
Srinivas Chitiveli, Offering Manager - PowerAI Vision, IBM Systems IBM
Medical practitioners are adopting AI embedded applications that diagnose diseases to ensure quick, accurate treatments for their patients. Current practices of developing AI applications can be complex and resource intensive. IBM PowerAI Vision,
empowers Radiologists to label datasets, train and even deploy models to analyze images and videos helping pinpoint anomalies. Attend this session to see IBM PowerAI Vision firsthand.
12:30 Session Break
12:40 Luncheon Presentation (Sponsorship Opportunity Available) or Enjoy Lunch on Your Own
1:40 Session Break
1:50 Chairperson’s Remarks
Sanjay Joshi, Chief of Technology, Healthcare and Life Sciences, H2O.ai
1:55 On the Journey towards a New Assay Data Analysis Landscape
Joerg Degen, PhD, Project Leader Research Informatics, Roche
In pharmaceutical research, drug project teams depend on the availability, reliability, and interpretability of all relevant experimental results as they form the basis for many every day decisions to design, create and progress compounds through
the pipeline. Applications and workflows for capturing the diverse experimental results are tailored to the needs of the laboratories that run the experiments, leading to a dynamic and complex system landscape. Some of the biggest challenges
that arise in this context are linked to data integration and quality as well as comprehensive access to the data. While these circumstances are not new and may look straight forward to solve at first, they become more apparent and problematic
as data variety and complexity of questions in research are ever increasing. Another important factor is the steadily growing amount of results that are produced outside of the organization, i.e. in the context of collaborations with academia
or other industry partners. Over the past two years we have fundamentally modernized our landscape for assay data analysis in order to strengthen the ability of our scientists to access and interpret all relevant drug project data more efficiently,
and to collaborate more effectively. In this context, we have refactored some of our foundational systems and significantly increased our capabilities in the areas of data analysis and application of predictive models, e.g. using in-house
machine learning approaches. The data and capabilities are now integrated and made available to all researchers via a single, comprehensive platform. Recently we have started a programme to leverage this platform not just for small molecules
and peptides but also for therapeutic proteins and oligonucleotides.
2:25 AI and Health: A Data and Process Approach
Sanjay Joshi, Chief of Technology, Healthcare and Life Sciences, H2O.ai
There has been a lot of hype recently regarding AI in Health and Life Sciences. Sanjay will present an introduction to a use-case based approach -- he will cover the broad categories of financial, operational and clinical use-cases. The learning
objectives are practical, business-oriented Machine Learning and Deep Learning with specific focus on the data and processes involved. Summary results from two specific clinical use-cases will be presented.
2:55 Project Asaka, Harnessing Underutilized Computing Resources for Deep Learning
Jack Harwood, Distinguished Engineer Office, CTO, Dell EMC
Maximizing application performance through GPUs and FPGAs (aka ‘accelerators’) is not new for genomics workflows. Recent advances in machine and deep learning methods leverage the accelerators to operate on larger data sets in an ever
shrinking time span.
3:10 A Modern
Approach to Data Storage for Next Generation Sequencing & Medical Imaging
Peter Godman, Co-founder & CTO, Qumulo
File storage is a critical component of the life sciences research workflow. For researchers to be able to do their work, their storage must be able to scale to and handle billions of files efficiently. They must also be able to access their research
data from anywhere in the world. Learn how universal-scale file storage allows research organizations to manage massive, globally distributed file sets with ease.
3:25 Refreshment Break in the Exhibit Hall with Poster Viewing (Commonwealth Hall)
4:00 Chairperson
Sanjay Joshi, Chief of Technology, Healthcare and Life Sciences, H2O.ai
4:00 Enhancing Precision Wellness with Knowledge Graphs and Semantic Analytics
James Hendler, PhD, Tetherless World Chair of Computer, Web and Cognitive
Sciences; Director, RPI-IBM Center for Health Empowerment by Analytics, Learning and Semantics, Institute for Data Exploration and Applications, Rensselaer Polytechnic Institute
Today’s patients, clinicians and researchers have gone from a world of too little data to one of too much. Discovering relevant information, integrating it from multiple sources, deciding what to believe, and exploring alternative treatments
are all challenges that go beyond many of today’s medical support systems. In this talk, we explore how AI and machine learning can be used by healthcare providers and consumers to better understand and overcome health challenges.
4:30 Drug Discovery at Scale: Interpreting Biology with High-Dimensional Data
Peter McLean, PhD, Data Scientist & Computational Biologist, Recursion Pharmaceuticals
Recursion Pharmaceuticals leverages image-based cellular phenotyping for drug discovery by using computer vision and machine learning to turn biological questions into tractable data-science questions. Translating data science-derived conclusions
back into a biological or business framework introduces its own challenges. Here, I will introduce some of Recursion’s approaches for addressing the challenges around distilling computational models of high-dimensional cellular morphology
- across hundreds of disease and treated states - into interpretable, actionable data.
5:00 Towards
Tailor-Made Drugs with AI-Driven Drug Design
Ton van Daelen, PhD, BIOVIA
Enabling pharma, biotech, and agrichemical businesses to more efficiently produce safe, efficacious medicines and agents is key to improving productivity and competitiveness. Recent advances in machine learning methods and artificial intelligence
(AI) have shown great promise in bringing true automation to this process and the potential for these tools to finally become mainstream. By leveraging both existing in-house and publicly available assay data, predictive models can be
trained and then applied to rapidly design small molecule or biologics therapeutic starting points in silico against desired target, anti-target, safety and toxicity profiles in parallel. Once optimized, these in silico molecules are passed
to the lab to be created and tested. Results from each “Virtual-to-Lab” cycle are then used to assess and retrain the predictive models ahead of the next design round. By tightly coupling the design cycle with predictive models,
which are in turn, tightly coupled to available assay results, research organizations can efficiently leverage all available data far more effectively than is currently possible. In turn, this helps research organization discover drugs
and chemicals not only faster, but with a much increased chance of meeting regulatory, business, and market requirements for a new product.
5:15 Architecting for Success with Machine Learning Data Platforms
Kurt Kuckein, Director, Marketing, DDN Storage
Machine learning is being applied to many aspects of precision medicine. Organizations with a vision for the future will realize how the data at the heart of their ML initiatives will require extensive scaling. This presentation reviews key
considerations for creating and developing ML data platforms to ensure deeper insights, a shorter path to value, and capability for effortless scaling.
5:30 Best of Show Awards Reception in the Exhibit Hall with Poster Viewing (Commonwealth Hall)
7:00 – 10:00 Bio-IT World After Hours @Lawn on D
**Conference
Registration Required. Please bring your conference badge, wristband, and photo ID for entry.
Thursday, May 17
7:30 am Registration Open (Commonwealth Hall) and Morning Coffee (Foyer)
8:00 PLENARY KEYNOTE SESSION & AWARDS PROGRAM (Amphitheater & Harborview 2)
9:45 Coffee Break in the Exhibit Hall and Poster Competition Winners Announced (Commonwealth Hall)
10:30 Chairperson’s Remarks
Piyush Bansal, Senior Industry Analyst, Transformational Health, Frost & Sullivan
10:40 Machine Learning Approaches Applied to Biomedical Data for Patient Stratification and Decision Support
Kimberly Robasky, PhD, Senior Translational Scientist, Renaissance Computing Institute, University of North Carolina at Chapel Hill
Some estimates place the cost of bringing a drug to market at one billion U.S dollars and hence reducing the cost and length of clinical trials can indirectly lower healthcare costs. One source of clinical trial failure, lack of efficacy and
safety, could be mitigated through decision support for patient stratification. As part of the NIH-funded Biomedical Data Translator project, we are integrating multiple, previously disparate datasets, which is empowering investigators
with new tools for data-driving patient subtyping. For example, through the Data Translator project, we can combine clinical records with exposure data in support of powerful models for classification. We have implemented supervised and
unsupervised machine learning models on these data to create decision trees both for predicting patient outcomes and for clustering patients by phenotype and exposure to better understand patient disease and response. Here we will present
the results from supervised and unsupervised machine learning models trained to real world evidence (RWE) from asthma phenotypes curated in the Carolina Data Warehouse (CDW-H), combined with publicly available exposome data (e.g., PM2.5,
ozone).
11:10 Respect Individual Differences – Analytical Innovation in Personalized Medicine via Big Data
Ray Liu, PhD, Senior Director & Head, Statistical Innovation & Consultation, Takeda
Patients are not a single, homogenous group. Instead, patients are heterogeneous and respond differently even to the same drug treatment. Big data has the potential to fulfill the promise of personalized medicine. This presentation will focus
on recent analytical innovation using big data to match the right patient to the right drug at the right dose at the right time.
11:40 Machine Learning: An Essential Tool for Building Digital Biomarkers
Shyamal Patel, Senior Manager, Digital Medicine, Pfizer, Inc.
We live in an ecosystem of connected devices (e.g. wearables, smartphones and IoT systems). Sensors embedded in these devices, which we interact with on a daily basis, capture rich real-world information about our health and well-being. In
this talk, I will use case studies to illustrate how machine learning can be used as a powerful tool for tapping into these data streams to develop and deploy digital biomarkers at scale.
12:10 pm Session Break
12:20 Luncheon Presentation (Sponsorship Opportunity Available) or Enjoy Lunch on Your Own
1:20 Dessert Refreshment Break in the Exhibit Hall with Poster Viewing (Commonwealth Hall)
1:55 Chairperson’s Remarks
John Methot, Director, Health Informatics Architecture, Dana-Farber Cancer Institute
2:00 Disease Classification in the Era of Data-Intensive Medicine
Kanix Wang, PhD, Research Professional, Booth School of Business, Institute for Genomics & Systems Biology, University of Chicago
We used insurance claims for over one-third of the U.S. population to create a subset of 128,989 families (481,657 unique individuals). Using these data, we estimated the heritability and familial environmental patterns of 149 diseases. We
then computed the environmental and genetic disease classifications for a set of 29 complex diseases after inferring their pairwise genetic and environmental correlations.
2:30 Enviro-Geno-Pheno State Approach and State-Based Biomarkers for Differentiation, Prognosis, Subtypes, and Staging
Lei Xu, PhD, Director, Centre for Cognitive Machines and Computational Health; Zhiyuan Chair Professor, Department of Computer Science and Engineering, Shanghai Jiao Tong University
In the joint space of geno-measures, pheno-measures, and enviro-measures, one point represents a bio-system behavior and a subset of points that locate adjacently and share a common system status represents a ‘state.’ The system
is characterized by such states learned from samples. This enviro-geno-pheno state is considered a biomarker, indicating ‘health/normal’ versus ‘risk/abnormal’ together with its associated enviro-geno-pheno condition.
3:00 PANEL DISCUSSION: Can We Improve Breast Cancer Patient Outcomes through Artificial Intelligence?
Maya Said, ScD, President & CEO, Outcomes4me, Inc. (Moderator)
Panelists:
Regina Barzilay, PhD, MacArthur Fellow and Delta Electronics Professor, Massachusetts Institute of Technology (MIT) Department of Electrical Engineering and Computer Science; Member, Computer Science and Artificial Intelligence
Laboratory, MIT
Kevin Hughes, MD, Co-Director, Avon Breast Evaluation Program, Massachusetts General Hospital; Associate Professor of Surgery, Harvard Medical School; Medical Director, Bermuda Cancer Genetics Risk Assessment Clinic
Osama Rahma, MD, Assistant Professor of Medicine, Center For Immuno-Oncology, Dana-Farber Cancer Institute
Newly diagnosed cancer patients attempting to understand their treatment options face the overwhelming task of filtering an information deluge, much of which is irrelevant, outdated and occasionally inaccurate. Additionally, matching their
diagnosis to best-in-class treatments or potential clinical trials, while simultaneously learning to navigate an extremely complex healthcare system is daunting, even for the most highly trained physicians. We will explore various
platforms aimed at improving patient outcomes by leveraging technology to help educate, track, and connect patients with personalized resources while simultaneously working to improve the care continuum and the development of new treatments.
We will explore the nexus of healthcare networks and their IT systems, clinical decision-making and delivery, R&D, and patients, for whom we all create our innovation solutions. Attendees will be interested to understand how various
groups are working to increase value across the entire system by bringing laboratory, clinical and pharmaceutical science, real-world evidence and patient-reported data together with technology and artificial intelligence to solve
health challenges. These approaches offer the opportunity to generate deeper insights into how therapies perform in the real world and harness that understanding to improve efficiency, effectiveness, value, and ultimately, patient
care.
4:00 Conference Adjourns